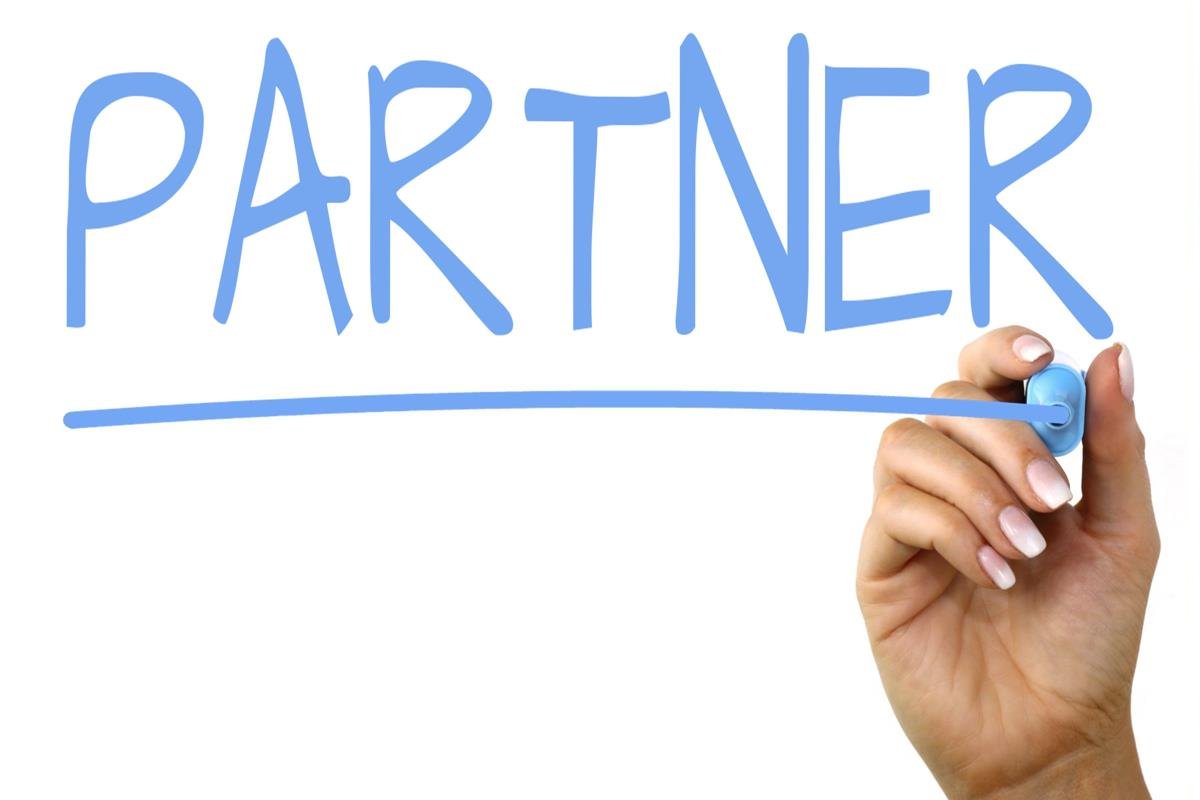
The Zdoc site has joined us so that our content creation team delivers even more quality to our users.
This is great news for GarminExpress, on another stage of growth!
Zdoc was a PDF free download professional platform.
On our site, you will find a variety of articles and news about casino games, betting, and cryptocurrencies!
Our articles are written by industry experts, who provide up-to-date and relevant information on the topics. You will find game reviews, betting strategies, tips for investing in cryptocurrencies, and more.
In addition, our site also has a news section, where you will stay up-to-date on the latest news in the world of gambling, betting, and cryptocurrencies.
Check out our site and stay up-to-date on everything that happens in the world of gambling, betting, and cryptocurrencies!
Here are some examples of articles you might find on our site:
- How to play blackjack and win money?
- The best strategies for sports betting
- How to invest in cryptocurrencies and not lose money
- The new law on gambling
- The future of sports betting with cryptocurrencies
Here are some examples of news you might find on our site:
- New sports betting site launches
- Cryptocurrency accepted as a form of payment at online casino
- Gambling law approved